[雪峰磁针石博客]计算机视觉opcencv工具深度学习快速实战1人脸识别
使用OpenCV提供的预先训练的深度学习面部检测器模型,可快速,准确的进行人脸识别。
2017年8月OpenCV 3.3正式发布,带来了高改进的“深度神经网络”(dnn deep neural networks)模块。该模块支持许多深度学习框架,包括Caffe,TensorFlow和Torch / PyTorch。
基于Caffe的面部检测器在这里。
需要两组文件:
- 定义模型体系结构的.prototxt文件
- .caffemodel文件,包含实际图层的权重
权重文件不包含在OpenCV示例目录。
OpenCV深度学习面部检测器如何工作?
# 模型下载:https://itbooks.pipipan.com/fs/18113597-320346529 # 代码存放:https://github.com/china-testing/python-api-tesing/tree/master/opencv_crash_deep_learning # 技术支持qq群144081101(代码和模型存放) # USAGE # python detect_faces.py --image rooster.jpg --prototxt deploy.prototxt.txt --model res10_300x300_ssd_iter_140000.caffemodel # import the necessary packages import numpy as np import argparse import cv2 # construct the argument parse and parse the arguments ap = argparse.ArgumentParser() ap.add_argument("-i", "--image", required=True, help="path to input image") ap.add_argument("-p", "--prototxt", required=True, help="path to Caffe 'deploy' prototxt file") ap.add_argument("-m", "--model", required=True, help="path to Caffe pre-trained model") ap.add_argument("-c", "--confidence", type=float, default=0.5, help="minimum probability to filter weak detections") args = vars(ap.parse_args()) # load our serialized model from disk print("[INFO] loading model...") net = cv2.dnn.readNetFromCaffe(args["prototxt"], args["model"]) # load the input image and construct an input blob for the image # by resizing to a fixed 300x300 pixels and then normalizing it image = cv2.imread(args["image"]) (h, w) = image.shape[:2] blob = cv2.dnn.blobFromImage(cv2.resize(image, (300, 300)), 1.0, (300, 300), (104.0, 177.0, 123.0)) # pass the blob through the network and obtain the detections and # predictions print("[INFO] computing object detections...") net.setInput(blob) detections = net.forward() # loop over the detections for i in range(0, detections.shape[2]): # extract the confidence (i.e., probability) associated with the # prediction confidence = detections[0, 0, i, 2] # filter out weak detections by ensuring the `confidence` is # greater than the minimum confidence if confidence > args["confidence"]: # compute the (x, y)-coordinates of the bounding box for the # object box = detections[0, 0, i, 3:7] * np.array([w, h, w, h]) (startX, startY, endX, endY) = box.astype("int") # draw the bounding box of the face along with the associated # probability text = "{:.2f}%".format(confidence * 100) y = startY - 10 if startY - 10 > 10 else startY + 10 cv2.rectangle(image, (startX, startY), (endX, endY), (0, 0, 255), 2) cv2.putText(image, text, (startX, y), cv2.FONT_HERSHEY_SIMPLEX, 0.45, (0, 0, 255), 2) # show the output image cv2.imshow("Output", image) cv2.waitKey(0)
执行:
$ python detect_faces.py --image rooster.jpg --prototxt deploy.prototxt.txt --model res10_300x300_ssd_iter_140000.caffemodel
上面的面部有74.30%的置信度。 尽管OpenCV的Haar级联因缺少“直接”角度的面孔,但通过使用OpenCV的深度学习面部探测器,依然能够测到脸部。
再来看三个面孔的示例:
python detect_faces.py --image iron_chic.jpg --prototxt deploy.prototxt.txt --model res10_300x300_ssd_iter_140000.caffemodel
视频,视频流和网络摄像头应用人脸检测
# USAGE # python detect_faces_video.py --prototxt deploy.prototxt.txt --model res10_300x300_ssd_iter_140000.caffemodel # import the necessary packages from imutils.video import VideoStream import numpy as np import argparse import imutils import time import cv2 # construct the argument parse and parse the arguments ap = argparse.ArgumentParser() ap.add_argument("-p", "--prototxt", required=True, help="path to Caffe 'deploy' prototxt file") ap.add_argument("-m", "--model", required=True, help="path to Caffe pre-trained model") ap.add_argument("-c", "--confidence", type=float, default=0.5, help="minimum probability to filter weak detections") args = vars(ap.parse_args()) # load our serialized model from disk print("[INFO] loading model...") net = cv2.dnn.readNetFromCaffe(args["prototxt"], args["model"]) # initialize the video stream and allow the cammera sensor to warmup print("[INFO] starting video stream...") vs = VideoStream(src=0).start() time.sleep(2.0) # loop over the frames from the video stream while True: # grab the frame from the threaded video stream and resize it # to have a maximum width of 400 pixels frame = vs.read() frame = imutils.resize(frame, width=400) # grab the frame dimensions and convert it to a blob (h, w) = frame.shape[:2] blob = cv2.dnn.blobFromImage(cv2.resize(frame, (300, 300)), 1.0, (300, 300), (104.0, 177.0, 123.0)) # pass the blob through the network and obtain the detections and # predictions net.setInput(blob) detections = net.forward() # loop over the detections for i in range(0, detections.shape[2]): # extract the confidence (i.e., probability) associated with the # prediction confidence = detections[0, 0, i, 2] # filter out weak detections by ensuring the `confidence` is # greater than the minimum confidence if confidence < args["confidence"]: continue # compute the (x, y)-coordinates of the bounding box for the # object box = detections[0, 0, i, 3:7] * np.array([w, h, w, h]) (startX, startY, endX, endY) = box.astype("int") # draw the bounding box of the face along with the associated # probability text = "{:.2f}%".format(confidence * 100) y = startY - 10 if startY - 10 > 10 else startY + 10 cv2.rectangle(frame, (startX, startY), (endX, endY), (0, 0, 255), 2) cv2.putText(frame, text, (startX, y), cv2.FONT_HERSHEY_SIMPLEX, 0.45, (0, 0, 255), 2) # show the output frame cv2.imshow("Frame", frame) key = cv2.waitKey(1) & 0xFF # if the `q` key was pressed, break from the loop if key == ord("q"): break # do a bit of cleanup cv2.destroyAllWindows() vs.stop()
执行:
python detect_faces_video.py --prototxt deploy.prototxt.txt --model res10_300x300_ssd_iter_140000.caffemodel
参考资料
- 本文最新版本地址
- 本文涉及的python测试开发库 谢谢点赞!
- 本文相关海量书籍下载
- 2018最佳人工智能机器学习工具书及下载(持续更新)
- 模型下载:https://itbooks.pipipan.com/fs/18113597-320346529
其他python人脸识别库介绍
python库介绍-face_recognition 人脸识别
可以命令识别人脸框。
$ face_detection --model cnn iron_chic.jpg iron_chic.jpg,79,422,243,258 iron_chic.jpg,146,272,310,108 iron_chic.jpg,194,144,330,7
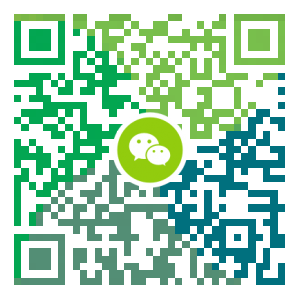
低调大师中文资讯倾力打造互联网数据资讯、行业资源、电子商务、移动互联网、网络营销平台。
持续更新报道IT业界、互联网、市场资讯、驱动更新,是最及时权威的产业资讯及硬件资讯报道平台。
转载内容版权归作者及来源网站所有,本站原创内容转载请注明来源。
- 上一篇
NLP-Progress记录NLP最新数据集、论文和代码: 助你紧跟NLP前沿
方向是自然语言处理的同学们有福啦,为了跟踪自然语言处理(NLP)的进展,有大量仁人志士在 Github 上维护了一个名为 NLP-Progress 的库。它记录了几乎所有NLP任务的 baseline 和 标准数据集,同时还记录了这些问题的state-of-the-art。 ●Github ●https://github.com/sebastianruder/NLP-progress ●官方网址 ●https://nlpprogress.com/ ●整理报道 ●huaiwen NLP-Progress 同时涵盖了传统的NLP任务,如依赖解析和词性标注,和一些新的任务,如阅读理解和自然语言推理。它的不仅为读者提供这些任务的 baseline 和 标准数据集,还记录了这些问题的state-of-the-art。 下面小编简单列举了几个NLP-Progress 记录的任务: ●Coreference resolution 共指消解 ●Dependency parsing 依存分析 ●Dialogue 对话 ●Domain Adaption 领域迁移 ●Entity Linking 实...
- 下一篇
Spring Boot中对自然语言处理工具包hanlp的调用详解
概 述 HanLP 是基于 Java开发的 NLP工具包,由一系列模型与算法组成,目标是普及自然语言处理在生产环境中的应用。而且 HanLP具备功能完善、性能高效、架构清晰、语料时新、可自定义的特点,因此十分好上手,本文就结合 Spring Boot来将 HanLP用起来! 下载 HanLP数据和程序 由于 HanLP库将数据与代码分离,因此我们需要分别下载所需数据和 jar包: (1)所需 data数据包下载地址为 data.zip (2)所需 jar包下载地址为 hanlp-release.zip 工程搭建 (1)创建一个普通的 Spring Boot工程,不赘述 (2)引入 HanLP数据 和 配置 下载完成以后,首先解压 hanlp-release.zip压缩包,然后将解压出的 HanLP的 jar包引入 Spring Boot工程,然后需要来放置 HanLP所需配置和数据: (1)将解压后 hanlp-release.zip压缩包中的 hanlp.properties配置文件置于项目的 resources资源目录下 (2)然后解压 data.zip压缩包,将解压出的 data...
相关文章
文章评论
共有0条评论来说两句吧...
文章二维码
点击排行
推荐阅读
最新文章
- CentOS7安装Docker,走上虚拟化容器引擎之路
- Docker快速安装Oracle11G,搭建oracle11g学习环境
- Windows10,CentOS7,CentOS8安装Nodejs环境
- Linux系统CentOS6、CentOS7手动修改IP地址
- SpringBoot2整合Thymeleaf,官方推荐html解决方案
- Docker使用Oracle官方镜像安装(12C,18C,19C)
- CentOS7编译安装Cmake3.16.3,解决mysql等软件编译问题
- CentOS7,8上快速安装Gitea,搭建Git服务器
- CentOS8编译安装MySQL8.0.19
- Jdk安装(Linux,MacOS,Windows),包含三大操作系统的最全安装