背包九讲问题
引言
通过学习背包九讲这个文档,掌握动态规划题目的解决方法。
1 背包问题
有N 件物品和一个容量为V 的背包。第i 件物品的费用(体积)是c[i],价值是w[i]。
求解将哪些物品装入背包可使价值总和最大。这里每一件物品只能取一次
1.1 思路
根据子问题定义状态,找出状态转移方程。
子问题就是:第i件物品是否放入背包。如果不放,那么第i件物品放入背包中的总价值和第i-1件物品放入背包的总价值相当。如果放入背包,也就是求出第i-1件物品放入v-c[i]的背包中时的值与第i件物品的价值的和,得到的就是总价值。
fi=max{fi-1,fi-1]+w[i]}
核心代码如下:
以下代码中,注意i和j的起始遍历位置,从第1行和第1列开始,此时的1表示的就是物品的编号。
//traverse N goods for(int i = 1;i<=N;i++){ for(int j = 1;j<=V;j++){ if(j-C[i]>=0){ f[i][j] = Math.max(f[i-1][j],f[i-1][j-C[i]]+W[i]); }else{ f[i][j] = f[i-1][j]; //这行代码可以不要。 } } }
1.2 初始化问题
已知有物品4件(N=4),背包容量V=10,每件物品的体积为5,4,6,3,每件物品的对应价值为10,40,30,50。
首先是关于初始化的问题,可以建立一个$(N+1)*(V+1)$的二维数组,增设第一行第一列是为了循环遍历的方便。一步步构建如下所示,初始时可以构建二维数组如下:
V N | 0 | 1 | 2 | 3 | 4 | 5 | 6 | 7 | 8 | 9 | 10 |
---|---|---|---|---|---|---|---|---|---|---|---|
0 | 0 | 0 | 0 | 0 | 0 | 0 | 0 | 0 | 0 | 0 | 0 |
1 | 0 | 0 | 0 | 0 | 0 | 0 | 0 | 0 | 0 | 0 | 0 |
2 | 0 | 0 | 0 | 0 | 0 | 0 | 0 | 0 | 0 | 0 | 0 |
3 | 0 | 0 | 0 | 0 | 0 | 0 | 0 | 0 | 0 | 0 | 0 |
4 | 0 | 0 | 0 | 0 | 0 | 0 | 0 | 0 | 0 | 0 | 0 |
得到的结果如下:
如果没有要求必须恰好装满背包,那么初始化的时候可以将二维数组全部初始化为0。若要求恰好装满背包,那么在初始化的时候除了第一行和第一列为0,其余均为无穷大。此时表最终的结果如下:
V N | 0 | 1 | 2 | 3 | 4 | 5 | 6 | 7 | 8 | 9 | 10 |
---|---|---|---|---|---|---|---|---|---|---|---|
0 | 0 | 0 | 0 | 0 | 0 | 0 | 0 | 0 | 0 | 0 | 0 |
1 | 0 | inf | inf | inf | inf | 10 | 10 | 10 | 10 | 10 | 10 |
2 | 0 | inf | inf | inf | 40 | 10 | 10 | 10 | 10 | 50 | 50 |
3 | 0 | inf | inf | inf | inf | inf | 30 | 10 | 10 | 50 | 70 |
4 | 0 | inf | inf | 50 | inf | inf | 30 | 10 | 10 | 80 | 70 |
需要注意的是,此时上述的核心代码中就要有一定的调整,调整如下:
//traverse N goods for(int i = 1;i<=N;i++){ for(int j = 1;j<=V;j++){ if(j-C[i]>=0){ f[i][j] = Math.max(f[i-1][j],f[i-1][j-C[i]]+W[i]); }else{ //这里的语句需要删掉,否则初始化为负无穷大就没有了意义。 } } }
1.3 被选择的物品
当求解出最大价值的时候,如何求出所选择的的物品?根据状态转移方程可知,当fi-1 = fi的时候,表示i没有放入背包,否则表示放入背包,此时减去该背包的体积,然后再判断该体积下的情况,直到第一件物品。
for(int i = N;i>=1;i--){ if(f[i-1][V] != f[i][V]){ System.out.print(i+" "); V -= C[i]; } }
1.4 空间优化
每一次的fi的值只与fi-1的值有关,因此可以修改为一维数组,f[v]表示将前i个物品装入容量为v的背包时的最大价值。主要是注意遍历的时候,第二个是倒序,此时表示每一次循环的时候,上一次保存的f[v]还没有发生变化,从而说明一件物品被使用了一次。如果不是倒序还是使用正序,此时会发现可以重复选择物品。
下面代码中打印输出了每一次循环的时候f[v]的值。使用一维数组实现,从而在空间上进行了优化。如果要实现恰好装满背包,那么初始化的时候,f[0]为0,其余初始化为无穷大。
for(int i = 1;i<=N;i++){ for(int j = V;j>=0;j--){ if(j>=C[i]){ f[j] = Math.max(f[j], f[j-C[i]]+W[i]); } } for(int j = 0;j<=V;j++){ System.out.print(f[j]+" "); } System.out.println(); }
1.5 使用二维数组解决背包问题
代码如下,注意其中两种的同的初始化。对于恰好装满背包问题,初始化数组的时候可以这样理解:初始化数据也就是初始化背包状态,即没有任何物品装入的时候的背包状态。如果要求背包恰好装满,那么最开始的时候只有背包容量为0的时候才有可能被价值为0的nothing恰好装满(因为背包的初始状态时什么也没装),如果容量大于1,那么此时因为背包什么也没装,则没有合法的解,属于未定义状态,可以初始化为$\inf$
# 0-1 knapsack problem: two-dimensional array and one-dimensional array are used to resolve this problem import numpy as np def knapsack_2_array(N, V, C, W): """ :param N: the number of goods :param V: the total volume of goods :param C: the volume of each goods type-list :param W: the weight of each goods type-list :return: f[N][V] """ # initialization # f = np.zeros((N+1, V+1)) # another initialization for just full of knapsack f = np.zeros((N+1, V+1)) for i in range(N+1): for j in range(V+1): if j == 0: f[i][j] = 0 else: f[i][j] = float('-inf') print(f) # repeat for i in range(1, N+1): for j in range(1, V+1): if j-C[i]>=0: f[i][j] = max(f[i-1][j], f[i-1][j-C[i]]+W[i]) else: f[i][j] = f[i-1][j] return f def get_choosed_goods_2_array(N, V, C, W, f): for i in range(N, 0, -1): if f[i][V] == f[i-1][V]: continue else: V = V-C[i] print(i) if __name__ == '__main__': N = 5 V = 10 C = [0, 6, 4, 4, 2, 3] W = [0, 8, 10, 4, 5, 5] f1 = knapsack_2_array(N, V, C, W) print(f1)
恰好装满时的初始化结果和运行结果 [[ 0. -inf -inf -inf -inf -inf -inf -inf -inf -inf -inf] [ 0. -inf -inf -inf -inf -inf -inf -inf -inf -inf -inf] [ 0. -inf -inf -inf -inf -inf -inf -inf -inf -inf -inf] [ 0. -inf -inf -inf -inf -inf -inf -inf -inf -inf -inf] [ 0. -inf -inf -inf -inf -inf -inf -inf -inf -inf -inf] [ 0. -inf -inf -inf -inf -inf -inf -inf -inf -inf -inf]] [[ 0. -inf -inf -inf -inf -inf -inf -inf -inf -inf -inf] [ 0. -inf -inf -inf -inf -inf 8. -inf -inf -inf -inf] [ 0. -inf -inf -inf 10. -inf 8. -inf -inf -inf 18.] [ 0. -inf -inf -inf 10. -inf 8. -inf 14. -inf 18.] [ 0. -inf 5. -inf 10. -inf 15. -inf 14. -inf 19.] [ 0. -inf 5. 5. 10. 10. 15. 15. 14. 20. 19.]] 被选择物品 4 3 2 没有要求恰好装满时的运行结果 [[ 0. 0. 0. 0. 0. 0. 0. 0. 0. 0. 0.] [ 0. 0. 0. 0. 0. 0. 8. 8. 8. 8. 8.] [ 0. 0. 0. 0. 10. 10. 10. 10. 10. 10. 18.] [ 0. 0. 0. 0. 10. 10. 10. 10. 14. 14. 18.] [ 0. 0. 5. 5. 10. 10. 15. 15. 15. 15. 19.] [ 0. 0. 5. 5. 10. 10. 15. 15. 15. 20. 20.]] 输出被选择的物品 5 4 2
1.6 使用一维数组解决问题
def knapsack_1_array(N, V, C, W): # initialization f = np.zeros(V+1) # initialization for just full of knapsack # f = np.zeros(V+1) # for i in range(V+1): # if i == 0: # f[i] = 0 # else: # f[i] = float('-inf') print("Output intermediate process result") for i in range(1, N+1): for j in range(V, 0, -1): if j-C[i] >= 0: f[j] = max(f[j], f[j-C[i]]+W[i]) print(f) print("Output the final result") return f def get_choosed_goods_1_array(N, V, C, W, f): for i in range(N, 0, -1): if f[V] == f[V-C[i]]+W[i]: V = V-C[i] print(i)
输出结果 [ 0. -inf -inf -inf -inf -inf -inf -inf -inf -inf -inf] Output intermediate process result [ 0. -inf -inf -inf -inf -inf 8. -inf -inf -inf -inf] [ 0. -inf -inf -inf 10. -inf 8. -inf -inf -inf 18.] [ 0. -inf -inf -inf 10. -inf 8. -inf 14. -inf 18.] [ 0. -inf 5. -inf 10. -inf 15. -inf 14. -inf 19.] [ 0. -inf 5. 5. 10. 10. 15. 15. 14. 20. 19.] Output the final result [ 0. -inf 5. 5. 10. 10. 15. 15. 14. 20. 19.] 4 3 2 没有要求恰好装满的时候 Output intermediate process result [0. 0. 0. 0. 0. 0. 8. 8. 8. 8. 8.] [ 0. 0. 0. 0. 10. 10. 10. 10. 10. 10. 18.] [ 0. 0. 0. 0. 10. 10. 10. 10. 14. 14. 18.] [ 0. 0. 5. 5. 10. 10. 15. 15. 15. 15. 19.] [ 0. 0. 5. 5. 10. 10. 15. 15. 15. 20. 20.] Output the final result [ 0. 0. 5. 5. 10. 10. 15. 15. 15. 20. 20.] 选择的物品 5 4 2
关于上面在j遍历的使用使用逆序的原因,下面举一个例子:如果按照j顺序遍历,当i=1,j=1,2的时候,f[1]=f[2] = 0;当j=3的时候,f[3] = max(f[3], f[3-3]+w[1])= max(0,4) = 4;当j=4,5的时候,f[4]=[5] = 4。但是当j=6的时候,有f[6] = max(f[6], f[6-3]+w[1]) = max(0, 8)。这个8其实是因为第一件物品取了两次得到的,这显然不符合01背包问题(每件物品只能取一次)
在输出结果的时候,注意遍历的方向,N是从最大值向最小值遍历,因为所得数组的最后一项内容是要求解的结果,所以需要从后向前遍历
2 完全背包问题
与0-1背包问题的差别就是每一个物品可以重复多次放入,因此子问题就相应的需要改变,针对第i个物品,不是放入背包或者不放入背包,而应该是放入0次,或者多次。因此状态转移方程需要进行修改如下:
$$ f[i][v]=max{f[i-1][v-k*c[i]]+k*w[i]|0<=k*c[i]<=v} $$
k值表示放入的次数。
import numpy as np def getMaxWorth(amount, total_capacity, volumes, worths): """ amount: the quantity of things type-int total_capacity: the volume of backpack type-int volumes: the volume of each thing type-list worths: the worth of each thing type-list """ # create a amount*total_capacity array and initialization f = np.zeros((amount+1,total_capacity+1), dtype=int) # repeat for i in range(1,amount+1): for j in range(1,total_capacity+1): temp = [] k = 0 while k*volumes[i] <= j : temp.append(f[i-1][j-k*volumes[i]]+k*worths[i]) k+=1 f[i][j] = max(temp) return f amount = 4 total_capacity=10 volumes=[0,5,4,6,3] worths=[0,10,40,30,50] getMaxWorth(amount, total_capacity, volumes, worths)
array([[ 0, 0, 0, 0, 0, 0, 0, 0, 0, 0, 0], [ 0, 0, 0, 0, 0, 10, 10, 10, 10, 10, 20], [ 0, 0, 0, 0, 40, 40, 40, 40, 80, 80, 80], [ 0, 0, 0, 0, 40, 40, 40, 40, 80, 80, 80], [ 0, 0, 0, 50, 50, 50, 100, 100, 100, 150, 150]])
上面的时间复杂度分析:此问题和01背包问题一样,有$O(N\*V)$个状态需要求解,但是每一个状态求解的时间已经发生了变化,求解状态fi的时间为O(v/c[i]),总的时间复杂度超过了O(NV),其中N是物品数量,V是背包容量。
2.1 简单优化方案
若两件物品i,j满足 volumes[i]< volumes[j] 并且 worths[i]>worths[j],则说明i物品体积小并且价值高,可以替换物品j,也就是可以将物品j删除掉。
import numpy as np def get_can_be_delete_item_index(volumes, worths): """ delete items with small volume and small value """ index_for_delete = set() for i in range(1, len(volumes)-1): for j in range(i+1, len(volumes)): if volumes[i] > volumes[j] and worths[i] < worths[j]: index_for_delete.add(i) return index_for_delete def getMaxWorth(amount, total_capacity, volumes, worths): """ amount: the quantity of things type-int total_capacity: the volume of backpack type-int volumes: the volume of each thing type-list worths: the worth of each thing type-list """ new_volumes = [] new_worths = [] index_delete = get_can_be_delete_item_index(volumes, worths) for i in range(0, len(volumes)): if i not in index_delete: new_volumes.append(volumes[i]) new_worths.append(worths[i]) # print(new_volumes, new_worths) amount = len(new_volumes) # create a amount*total_capacity array and initialization f = np.zeros((amount,total_capacity+1), dtype=int) volumes = new_volumes worths = new_worths # repeat for i in range(1,amount): for j in range(1,total_capacity+1): temp = [] k = 0 while k*volumes[i] <= j : temp.append(f[i-1][j-k*volumes[i]]+k*worths[i]) k+=1 f[i][j] = max(temp) return f amount = 4 total_capacity=10 volumes=[0,5,4,6,3] worths=[0,10,40,30,50] getMaxWorth(amount, total_capacity, volumes, worths)
array([[ 0, 0, 0, 0, 0, 0, 0, 0, 0, 0, 0], [ 0, 0, 0, 50, 50, 50, 100, 100, 100, 150, 150]])
此问题还可以试着输出一下被选择的物品和次数之间的关系,以及如何选择物品可以恰好装满
3 多重背包问题
在问题的基础上,增加每件物品的件数,比如,第i种物品最多n[i]件可用。
转移状态方程:共有N种物品,假设fi-1是将前i-1种物品放入容量v中时的最大价值,那么针对第i种物品,它最多可以放入n[i]次,此时的转义方程如下:
$$ f[i][v] = max(f[i-1][v-k*c[i]]+k*w[i] | 0<=k<=n[i]) $$
因此,在上面代码的基础上做了一些改变,如下。其中需要注意在逆推得到每件物品的时候,从最后一种物品开始,体积是从V开始并且不是遍历V,而是根据结果动态改变计算出下一次的V
import numpy as np def getMaxWorth(amount, total_capacity, volumes, worths, counts): """ amount: the quantity of things type-int total_capacity: the volume of backpack type-int volumes: the volume of each thing type-list worths: the worth of each thing type-list counts: the number of each thing type-list """ # create a amount*total_capacity array and initialization f = np.zeros((amount + 1, total_capacity + 1), dtype=int) # repeat for i in range(1, amount + 1): for j in range(1, total_capacity + 1): temp = [] k = 0 while k * volumes[i] <= j and k <= counts[i]: temp.append(f[i - 1][j - k * volumes[i]] + k * worths[i]) k += 1 f[i][j] = max(temp) return f def get_the_goods(f, amount, total_capacity, counts, volumes,worths): """ f: the f[i][v] amount: the number of things return: the goods and its choosed times """ flag = False for i in range(amount, 0, -1): flag = False for k in range(1, counts[i] + 1): if total_capacity-k*volumes[i] >= 0: # note: the point is to use the state transfer formula to compute the k value while f[i][total_capacity] == f[i - 1][total_capacity-k*volumes[i]] + k * worths[i]: total_capacity -= k * volumes[i] print("第%d件物品取%d件" % (i, k)) flag = True break if flag: break amount = 3 total_capacity = 8 volumes = [0, 1,2,2] worths = [0, 6,10,20] counts = [0,10,5,2] f = getMaxWorth(amount, total_capacity, volumes, worths, counts) print(f) get_the_goods(f, amount, total_capacity, counts, volumes,worths)
输出 [[ 0 0 0 0 0 0 0 0 0] [ 0 6 12 18 24 30 36 42 48] [ 0 6 12 18 24 30 36 42 48] [ 0 6 20 26 40 46 52 58 64]] 第3件物品取2件 第1件物品取4件
根据以上代码可以发现,该算法的时间复杂度是$O(V \times \sum{counts[i]})$(时间复杂度计算:比如第一种物品的时候,会执行$V \times counts[1]$次,第二种物品,程序会执行$V \times counts[2]$次,因此可以得到总的时间复杂度)可以发现时间复杂度与物品的次数有关。
该方法可以转化成01背包问题进行思考,就是将第i种物品,如果出现次数为n[i],此时可以得到一个包含有$\sum{n[i]}$中物品的01背包问题,此时的时间复杂度仍然是$O(V \times \sum{counts[i]})$
方法:将第i种物品分成若干件物品,其中每件物品有一个系数,这件物品的费用和价值是原来的物品的费用和价值乘以这个系数,使这些系数分别为$1,2,4,...2^{(k-1)}$,其中k应该满足 $n[i]-2^k+1>0$的最大整数,比如,n[i]=13,那么k最大为3,从而得到1,2,4;之后使用n[i]-(1+2+4)得到6,因此可以将这个物品的系数分为1,2,4,6;这样的系数可以表示0~n[i]中的每一个整数,同时n[i]件物品对应的就变成了$log(n[i])$件物品,事件复杂度为$O(V \times \sum{log({n[i]}))}$。
其中,将背包中对应数量的物品拆分成指定系数的物品代码如下:
def multiple_pack(volumes, worths, counts): """ make the count of goods to different v and w goods :param volumes: list :param worths: list :param counts: list :return: tuple(list,list,list) """ v = [0] w = [0] for i in range(1, len(counts)): # get the max value k according to the counts[i] k = int(math.sqrt(counts[i])) # generate the coeffcient according to k k_list = [math.pow(2,item) for item in range(k)] k_list.append(counts[i]-sum(k_list)) for item in k_list: v.append(int(item * volumes[i])) w.append(int(item * worths[i])) return (v, w)
输入 volumes = [0, 1, 2, 2] worths = [0, 6, 10, 20] counts = [0, 10, 5, 2] 输出 [0, 1, 2, 4, 3, 2, 4, 4, 2, 2] [0, 6, 12, 24, 18, 10, 20, 20, 20, 20]
之后使用01背包问题进行求解,代码如下所示:
def get_f_of_01_backpack(n, total_capacity, volumes, worths): """ :param n: the number of goods :param volumes: list :param worths: list :return: f """ # initialization f = np.zeros((n+1, total_capacity+1)) #repeat for i in range(1, n+1): for j in range(1, total_capacity+1): if j-volumes[i] >= 0: f[i][j] = max(f[i-1][j], f[i-1][j-volumes[i]]+worths[i]) else: f[i][j] = f[i-1][j] return f
输入 n = 9 total_capacity = 8 volumes = [0, 1, 2, 4, 3, 2, 4, 4, 2, 2] worths = [0, 6, 12, 24, 18, 10, 20, 20, 20, 20] 输出 [[ 0. 0. 0. 0. 0. 0. 0. 0. 0.] [ 0. 6. 6. 6. 6. 6. 6. 6. 6.] [ 0. 6. 12. 18. 18. 18. 18. 18. 18.] [ 0. 6. 12. 18. 24. 30. 36. 42. 42.] [ 0. 6. 12. 18. 24. 30. 36. 42. 48.] [ 0. 6. 12. 18. 24. 30. 36. 42. 48.] [ 0. 6. 12. 18. 24. 30. 36. 42. 48.] [ 0. 6. 12. 18. 24. 30. 36. 42. 48.] [ 0. 6. 20. 26. 32. 38. 44. 50. 56.] [ 0. 6. 20. 26. 40. 46. 52. 58. 64.]]
通过逆向推导,可以得到被选择的物品。从最后一个物品开始遍历,直到到达第一个物品,需要注意的是提及V是根据结果进行动态调整的,最开始应该是体积V。
def get_path_of_01_backpack(n,total_capacity, volumes, f): for i in range(n, 0,-1): if f[i][total_capacity] != f[i-1][total_capacity]: total_capacity -= volumes[i] print(i)
输出 9 8 3
与上面的第一种方法进行对比可以看出结果是一样的,选择的东西也是一样的,只不过一个是拆开的物品。
本小结主要是关于将算法的复杂度进行改进,需要特别注意的是“拆分物品”的思想和方法。
4 混合三种背包问题
将前面三种背包的问题混合起来,也就是有的物品可以取一次(01背包),有的物品可以无限次取(完全背包),有的物品可以取有限次(多重背包问题)。应该怎么求解。我的思路就是有一个存储背包次数的数组,如果可取有限次,就对应的是物品的次数,如果是无限次,就先根据物品的体积和总体积得到每件物品的最大可取次数并填入数组中。
5 二维费用的背包问题
对于每件物品,具有两种不同的费用:选择这件物品的时候必须同时付出两种代价,对于每种代价都有一个可以付出的最大值(比如背包容量V和最多可取物品数量M),问怎样选择物品可以达到最大价值。设第i件物品的两种代价分别为a[i]和b[i],两种代价的最大值为V,M。
思路:费用增加一维,只需状态增加一维即可.设fi[m]表示前i件物品付出v和m代价时的最大价值,那么状态转移方程如下:
$$ f[i][v][m] = max{f[i-1][v][m], f[i-1][v-a[i]][m-b[i]]+w[i]} $$
当然,和前面的方法一样,也能使用二维数组来解决:当每件物品只可以取一次的时候,变量v,m逆序循环,当物品可以取无限次的时候,可以采用顺序的循环。当物品有如多重背包问题的时候,可以拆分物品,然后使用01背包问题求解。
5.1 使用三维数组解决问题
# 2-dimensional cost knapsack problem # 0-1 knapsack problem: two-dimensional array and one-dimensional array are used to resolve this problem import numpy as np def knapsack_2_array(N, V, M, A, B, W): """ :param N: the number of goods :param V: the total volume1 of goods :param M: the total volume2 of goods :param A: the volume1 of each goods type-list :param B: the volume2 of each goods type-list :param W: the weight of each goods type-list :return: """ # initialization f = np.zeros((V+1, M+1)) # initialization for just full of M # f = np.zeros((V+1, M+1)) # for i in range(V+1): # for j in range(M+1): # if j == 0: # f[i][j] = 0 # else: # f[i][j] = float('-inf') # repeat for i in range(1, N+1): for j in range(V, 0,-1): for k in range(M, 0, -1): if j-A[i] >= 0 and k-B[i] >= 0: f[j][k] = max(f[j][k], f[j-A[i]][k-B[i]]+W[i]) # print(f) return f def get_choosed_goods(N, V, M, A, B, W, f): for i in range(N, 0, -1): if f[V][M] == f[V-A[i]][M-B[i]]+W[i] and f[V][M] != float('-inf') and f[V-A[i]][M-B[i]] != float('-inf'): V -= A[i] M -= B[i] print(i) if __name__ == '__main__': N = 5 V = 10 M = 10 A = [0,6, 4, 2, 2, 3] B = [0, 8,4,2,2,3] W = [0,8,10,4,5,5] f1 = knapsack_2_array(N, V, M, A, B, W) print(f1)
如果没有要求背包恰好装满,初始化的时候全部初始化为0即可,那么输出结果是: [[ 0. 0. 0. 0. 0. 0. 0. 0. 0. 0. 0.] [ 0. 0. 0. 0. 0. 0. 0. 0. 0. 0. 0.] [ 0. 0. 5. 5. 5. 5. 5. 5. 5. 5. 5.] [ 0. 0. 5. 5. 5. 5. 5. 5. 5. 5. 5.] [ 0. 0. 5. 5. 10. 10. 10. 10. 10. 10. 10.] [ 0. 0. 5. 5. 10. 10. 10. 10. 10. 10. 10.] [ 0. 0. 5. 5. 10. 10. 15. 15. 15. 15. 15.] [ 0. 0. 5. 5. 10. 10. 15. 15. 15. 15. 15.] [ 0. 0. 5. 5. 10. 10. 15. 15. 15. 15. 15.] [ 0. 0. 5. 5. 10. 10. 15. 15. 15. 20. 20.] [ 0. 0. 5. 5. 10. 10. 15. 15. 19. 20. 20.]] 5 4 2 如果要求恰好装满M,也就是M=0的这一列为0,其余位-inf,那么初始化的结果为: [[ 0. -inf -inf -inf -inf -inf -inf -inf -inf -inf -inf] [ 0. -inf -inf -inf -inf -inf -inf -inf -inf -inf -inf] [ 0. -inf -inf -inf -inf -inf -inf -inf -inf -inf -inf] [ 0. -inf -inf -inf -inf -inf -inf -inf -inf -inf -inf] [ 0. -inf -inf -inf -inf -inf -inf -inf -inf -inf -inf] [ 0. -inf -inf -inf -inf -inf -inf -inf -inf -inf -inf] [ 0. -inf -inf -inf -inf -inf -inf -inf -inf -inf -inf] [ 0. -inf -inf -inf -inf -inf -inf -inf -inf -inf -inf] [ 0. -inf -inf -inf -inf -inf -inf -inf -inf -inf -inf] [ 0. -inf -inf -inf -inf -inf -inf -inf -inf -inf -inf] [ 0. -inf -inf -inf -inf -inf -inf -inf -inf -inf -inf]] 输出结果 [[ 0. -inf -inf -inf -inf -inf -inf -inf -inf -inf -inf] [ 0. -inf -inf -inf -inf -inf -inf -inf -inf -inf -inf] [ 0. -inf 5. -inf -inf -inf -inf -inf -inf -inf -inf] [ 0. -inf 5. 5. -inf -inf -inf -inf -inf -inf -inf] [ 0. -inf 5. 5. 10. -inf -inf -inf -inf -inf -inf] [ 0. -inf 5. 5. 10. 10. -inf -inf -inf -inf -inf] [ 0. -inf 5. 5. 10. 10. 15. -inf 8. -inf -inf] [ 0. -inf 5. 5. 10. 10. 15. 15. 8. -inf -inf] [ 0. -inf 5. 5. 10. 10. 15. 15. 8. -inf 13.] [ 0. -inf 5. 5. 10. 10. 15. 15. 8. 20. 13.] [ 0. -inf 5. 5. 10. 10. 15. 15. 19. 20. 13.]] 被选择的物品 4 1
注意在输出被选择的物品的时候,是需要逆序输出的,同时需要注意存在float('-inf') = float('-inf')+10,也就是inf与一个数的和与inf相等,因此需要在输出的时候注意值是否为inf
思考:如何保证恰好满足V和M?该如何进行初始化??
6 分组的背包问题
有N件物品和一个容量为V的背包,第i件物品的体积和价值分别为c[i]和w[i]。这些物品被划分为若干组,每组中的物品相互冲突,最多选择一件。求解将哪些物品装入背包的时候可以使这些物品的体积总和不超过背包容量,且可以取得最大的价值。
思路:因为物品划分成了组,所以就以组作为索引,而不是物品。则问题可以描述为,针对第k组,选择本组中的某一件还是一件都不选。fk表示前k组在体积为v的时候取得的最大价值,那么状态转移方程为:
$$ f[k][v] = max{f[k-1][v], f[k-1][v-c[i]]+w[i]| i是第k组中的物品} $$
核心代码如下:
for k in 1-->K: for v in V-->0: # 对于k组中的每一个物品 for i in k: f[v] = max{f[v], f[v-c[i]]+w[i]}
分组背包问题可以使用完全背包问题中的提到的一个简单的优化方法。
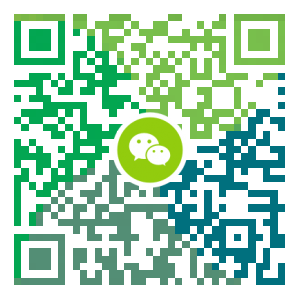
低调大师中文资讯倾力打造互联网数据资讯、行业资源、电子商务、移动互联网、网络营销平台。
持续更新报道IT业界、互联网、市场资讯、驱动更新,是最及时权威的产业资讯及硬件资讯报道平台。
转载内容版权归作者及来源网站所有,本站原创内容转载请注明来源。
- 上一篇
用阿里云函数计算部署thinkphp5.1
thinkphp国内还是用的很多的哈,我自己就用哈。所以研究了一下用函数计算部署tp5。其中借鉴和学习了@rsong 的文章:《十分钟上线-函数计算玩转 WordPress》 这里是TP5的演示:https://31199382.cn-beijing.fc.aliyuncs.com/2016-08-15/proxy/web-site/tp5/其实也没什么好演示的,就是纯净的tp5 ( ̄▽ ̄)~* 步骤: 1.后台创建php7.2环境的函数,创建http触发器,这些就不详细写了,可以去看文档。 2.打开tp5的入口文件(/public/index.php)在namespace think; 这句的下面,加如下代码: if(!$_SERVER['PATH_INFO']) $_SERVER['PATH_INFO'] = preg_replace("/^(\?s\=\/)/","",$_SERVER['REQUEST_URI']); 3.准备tp5源码,在根目录创建index.php文件(作为函数的入口文件),代码如下:(第一次在阿里云博客上写文章哈,有点手生) <?php #自定义的域...
- 下一篇
httpd编译安装php
wget http://hk1.php.net/distributions/php-5.6.31.tar.gz yum groupinstall "Development Tools" yum install zlib openssl perl yum install httpd httpd-devel tar -xf php.tar.gz -C /usr/src cd /usr/src/php yum install mariadb mariadb-server php-mysql 配置 ./configure --prefix /usr/local/php56 --with-apxs2=/usr/local/httpd24/bin/apxs --with-config-file-path=/etc/ --with-config-file-scan-dir=/etc/php.d --with-libxml-dir --with-openssl --with-zlib --enable-mbstring --with-mysql 其中php与httpd的联系就是apxs, 与mysql...
相关文章
文章评论
共有0条评论来说两句吧...
文章二维码
点击排行
推荐阅读
最新文章
- CentOS7安装Docker,走上虚拟化容器引擎之路
- SpringBoot2整合Thymeleaf,官方推荐html解决方案
- CentOS7设置SWAP分区,小内存服务器的救世主
- Hadoop3单机部署,实现最简伪集群
- CentOS8安装Docker,最新的服务器搭配容器使用
- CentOS8安装MyCat,轻松搞定数据库的读写分离、垂直分库、水平分库
- CentOS7编译安装Gcc9.2.0,解决mysql等软件编译问题
- Docker使用Oracle官方镜像安装(12C,18C,19C)
- CentOS7,8上快速安装Gitea,搭建Git服务器
- CentOS7编译安装Cmake3.16.3,解决mysql等软件编译问题